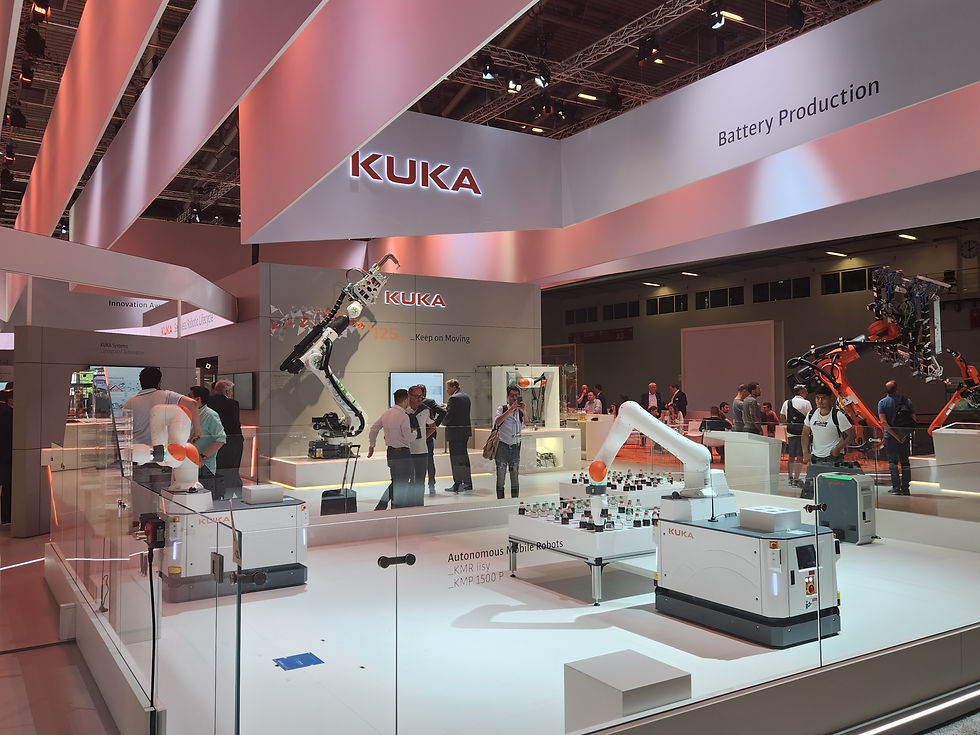
Unlocking Efficiency in Industry 4.0: The Role of Foundational Visibility in Robotic Systems Integration in the Context of Uptime and ROI
RSI Speaks Robot: A New Era of Automated Anomaly Detection
The advent of Industry 4.0 has brought about a significant shift in how industries operate, with a strong emphasis on automation and data exchange. One of the key technologies enabling this transformation is Robotic Systems Integration (RSI), a system that allows for seamless communication and interaction between various robotic components and systems.
Automated Anomaly Detection
Automated anomaly detection is a critical aspect of RSI. It involves using machine learning algorithms and statistical models to identify unusual patterns or behaviors in the data generated by robotic systems. This can help in the early detection of potential issues, thereby reducing downtime and improving operational efficiency1.
Foundational Visibility in Robotic Systems Integration
In the context of Robotic Systems Integration (RSI), foundational visibility is a crucial aspect that refers to the ability to monitor, track, and understand the performance and interactions of various robotic components and systems in real time. This visibility is foundational because it forms the basis for effective decision-making, troubleshooting, and system optimization.
Importance of Foundational Visibility
The importance of foundational visibility in RSI stems from modern industrial systems' complex and interconnected nature. These systems often involve many robotic components and systems, each performing specific tasks and interacting with each other in intricate ways. Identifying issues, optimizing performance, and making informed decisions can be easy with a clear understanding of these interactions and their impact on overall system performance. Foundational visibility allows for the real-time monitoring of various system parameters, such as operational status, performance metrics, and error logs. This information can be used to quickly identify and resolve issues, thereby reducing downtime and improving system reliability. Moreover, foundational visibility can provide valuable insights into system performance and efficiency. Analyzing the collected data makes it possible to identify trends, patterns, and areas for improvement. This can lead to more efficient operation, reduced costs, and improved productivity.
Achieving Foundational Visibility
Achieving foundational visibility in RSI involves the use of various tools and technologies. For instance, sensors can collect real-time data on different system parameters. This data can then be processed and analyzed using advanced analytics and machine learning algorithms to extract meaningful insights.
Intelligent Analysis in Robotic Systems Integration
Intelligent analysis is a critical component of Robotic Systems Integration (RSI). It enables organizations to make sense of the vast amounts of data their robotic systems generate and use it to drive decision-making and performance improvements.
What is Intelligent Analysis?
In the context of RSI, intelligent analysis refers to using advanced analytics techniques, machine learning, and artificial intelligence to analyze and interpret the data generated by various robotic systems. This can include data on system performance, operational status, error logs, and more.
Intelligent analysis aims to extract meaningful insights from this data that can be used to identify issues, optimize system performance, and make informed decisions. This can involve anomaly detection, predictive maintenance, root cause analysis, and system optimization.
The Importance of Intelligent Analysis
The importance of intelligent analysis in RSI cannot be overstated. Modern industrial systems are complex and generate vast amounts of data. Without intelligent analysis, making sense of this data and using it effectively can be challenging.
By providing a deep understanding of system performance and behavior, intelligent analysis can help organizations identify and resolve issues quickly, reducing downtime and improving system reliability. Moreover, it can provide valuable insights into system performance and efficiency, enabling organizations to optimize their operations and achieve higher levels of productivity and cost-effectiveness.
How Intelligent Analysis Works
Intelligent analysis in RSI typically involves several steps. First, data is collected from various robotic systems using sensors and other data collection devices. This data is then preprocessed to remove noise and prepare it for analysis.
Next, the preprocessed data is analyzed using advanced analytics techniques and machine learning algorithms. This can involve tasks such as anomaly detection, where unusual patterns in the data are identified that could indicate potential issues, and predictive maintenance, where machine learning models are used to predict when a component might fail based on historical data.
Finally, the analysis results are presented in a user-friendly format, such as a dashboard or report, enabling decision-makers to quickly understand the state of their systems and make informed decisions.
RSI also enables intelligent analysis of complex systems. By leveraging advanced analytics and machine learning techniques, RSI can help diagnose issues and identify the root cause of problems. This not only reduces troubleshooting time but also helps in improving the overall system performance.
Vendor Accountability
RSI metrics can track key performance indicators (KPIs) such as latency, response times, and disconnects. This can help hold vendors accountable and ensure they meet their service level agreements (SLAs). Moreover, these metrics can also provide valuable insights for improving system design and performance.
Outcomes
The use of RSI can lead to several positive outcomes. For instance, it can help in improving the visibility and uptime of robotic and industrial automation operations. Moreover, it can also aid in designing and implementing future factories and Industry 4.0 initiatives.
Advancing Automation
RSI plays a crucial role in advancing automation. Providing the necessary visibility and data allows innovation teams to move forward with greater confidence and speed. This can lead to the development of more efficient and effective automation solutions.
Time to Innocence
RSI can also help reduce the "time to innocence," i.e., the time it takes to prove that a particular component or system is not at fault. This can help avoid unnecessary troubleshooting efforts and focus on the actual root cause of the problem.
Uptime and ROI in the Context of Robotic Systems Integration
In industrial automation and robotic systems, uptime and return on investment (ROI) are two critical metrics that directly impact operations' efficiency, productivity, and profitability.
Uptime
Uptime is when a system or component is operational and available for use. In the context of Robotic Systems Integration (RSI), uptime measures the reliability and performance of integrated robotic systems. High uptime indicates that the systems function as expected with minimal disruptions or downtime.
The importance of uptime in RSI cannot be overstated. In an automated industrial environment, any downtime can lead to significant production delays, increased costs, and potential loss of revenue. Therefore, maintaining high uptime is crucial for any organization implementing RSI.
RSI can contribute to improved uptime in several ways. For instance, RSI's automated anomaly detection feature can help identify potential issues before they result in system failures, thereby preventing downtime. Similarly, the foundational visibility provided by RSI allows for real-time monitoring and control of robotic systems, which can further enhance system reliability and uptime.
Return on Investment (ROI)
Return on investment (ROI) is a financial metric widely used to measure the probability of a return on investment. It is a ratio that compares the gain or loss from an investment relative to its cost.
In the context of RSI, ROI can be viewed as the financial return obtained from the investment in robotic systems and their integration. This return can come in various forms, such as increased productivity, reduced operational costs, improved product quality, and enhanced customer satisfaction.
RSI can significantly contribute to improving ROI. RSI can increase productivity and reduce operational costs by enabling efficient operation and control of robotic systems, thereby boosting ROI. Moreover, the intelligent analysis capabilities of RSI can help optimize system performance and identify improvement areas, which can further enhance ROI.
In conclusion, uptime and ROI are two critical metrics in the context of RSI. RSI can significantly improve uptime and ROI by enabling automated anomaly detection, foundational visibility, and intelligent analysis, leading to more efficient and profitable operations.
Volkmar Kunerth
CEO
Accentec Technologies LLC & IoT Business Consultants
Schedule a meeting with me on Calendly: 15-min slot
Phone: +1 (650) 814-3266
Schedule a meeting with me on Calendly: 15-min slot
Check out our latest content on YouTube
Subscribe to my Newsletter, IoT & Beyond, on LinkedIn.
Sources
Chandola, V., Banerjee, A., & Kumar, V. (2009). Anomaly detection: A survey. ACM computing surveys (CSUR), 41(3), 1-58.
Wurman, P. R., D'Andrea, R., & Mountz, M. (2008). Coordinating hundreds of cooperative, autonomous vehicles in warehouses. AI magazine, 29(1), 9-9.
Ransing, R. S., & Lewis, R. W. (2001). A new approach to the rapid solution of inverse problems in flexible multibody systems. Proceedings of the Institution of Mechanical Engineers, Part K: Journal of Multi-body Dynamics, 215(3), 177-190.
Baccelli, F., Cohen, G., Olsder, G. J., & Quadrat, J. P. (1992). Synchronization and linearity: an algebra for discrete event systems. Wiley.
Lu, Y. (2017). Industry 4.0: A survey on technologies, applications and open research issues. Journal of Industrial Information Integration, 6, 1-10.
Wuest, T., Wee, D., & Lee, G. (2016). Extending discrete event simulation by adding an activity-based costing layer for increased decision support. International Journal of Production Economics, 182, 144-153.
Sipos, R., Fradkin, D., Moerchen, F., & Wang, Z. (2014, August). Log-based predictive maintenance. In Proceedings of the 20th ACM SIGKDD international conference on Knowledge discovery and data mining (pp. 1867-1876).
Lee, J., Lapira, E., Bagheri, B., & Kao, H. A. (2013). Recent advances and trends in predictive manufacturing systems in big data environments. Manufacturing Letters, 1(1), 38-41
#RoboticSystemsIntegration #IntelligentAnalysis #Industry4_0 #DataAnalytics #MachineLearning #ArtificialIntelligence #PredictiveMaintenance #OperationalEfficiency #DigitalTransformation #Innovation #TechnologyTrends #Automation #Robotics #BigData #IoT #AI #AdvancedAnalytics #IndustrialAutomation #SmartManufacturing #FutureOfWork"
Comments